Deep Learner: Building AI to Improve Cardiovascular Care
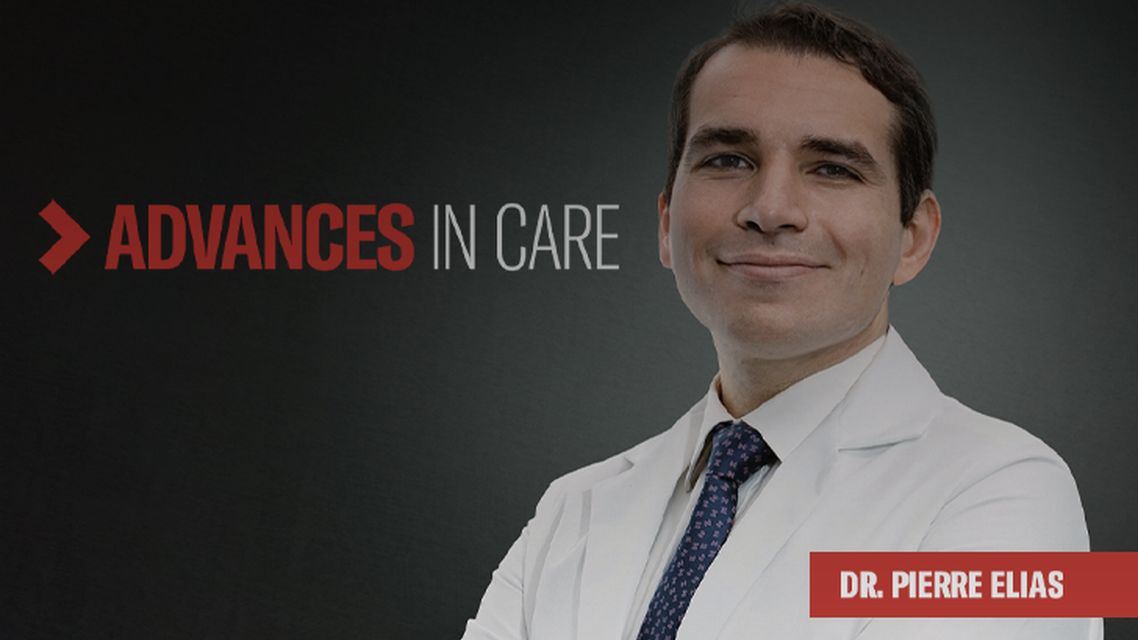
ReachMD Announcer:
Welcome to Advances in Care on ReachMD. This medical industry feature is titled “Deep Learner: Building AI to Improve Cardiovascular Care.” These podcasts are a production of NewYork-Presbyterian with doctors from Columbia & Weill Cornell Medicine.
Here are your guests, cardiologist Dr. Pierre Elias and health and science journalist, Catherine Price.
Catherine Price:
One day, Dr. Pierre Elias was walking down the hallway at Columbia University’s Irving Medical Center. When his colleague flagged him down and said, “You’re just the person I was looking for.”
Dr. Elias stopped, expecting his colleague to consult with him about a patient. But instead, she looked at him and said, “I can’t figure out how to get my new phone to connect to the hospital WIFI.”
Dr. Elias is used to his colleagues thinking of him as the go-to IT guy. But, in reality, he’s the Medical Director for Artificial Intelligence for NewYork-Presbyterian. What he actually does is a lot more complicated than managing WIFI passwords. He combines insights from the fields of technology, data science, and medicine, to build algorithms that help doctors provide better care for cardiac patients.
I’m Catherine Price, and this is Advances in Care.
On today’s episode Dr. Pierre Elias tells us why he believes that machine learning technologies will be key to the future of medicine, freeing up doctors to do what they do best: use their expertise to compassionately care for their patients.
First of all, it's great to meet you. Thank you so much for making the time to talk with us for this podcast.
Dr. Pierre Elias:
Sure. Thank you so much for having me.
Catherine Price:
And I wanted to start by just asking you to introduce yourself. Tell us who you are and what you do.
Dr. Pierre Elias:
My name's Pierre Elias. I'm an Assistant Professor in Cardiology and Biomedical informatics at Columbia University, and I'm also the Medical Director for Artificial Intelligence for New-York Presbyterian Hospital.
Catherine Price:
Can you tell me a bit about where things stand right now and the challenges that you currently see when it comes to the integration of medicine and technology?
Dr. Pierre Elias:
So I, you know, I'm able to find out someone is having a heart attack and put them on heart lung bypass and we can literally replace their organs for a period of time and help them recover.
Catherine Price:
Mm-hmm.
Dr. Pierre Elias:
And yet the way that I find out someone is having a heart attack in the hospital is I carry around a pager that tells me there’s some location in the hospital, someone's having a heart attack and I go there and say, “Hey, where's the person who's having a heart attack?” You know? When was the last time you sent a fax? I sent one, two weeks ago.
Catherine Price:
Yes. Seriously.
Dr. Pierre Elias:
Exactly. Medicine is this fascinating dichotomy of the most advanced technologies, the most ambitious things we as humans have ever attempted to do and stale tech from 30 years ago.
Catherine Price:
Okay.
Dr. Pierre Elias:
It feels like technology is a hurdle that we have to often overcome.
There's this picture, it's a child's drawing that was published in the Journal of the American Medical Association, JAMA.
And in this drawing you see the little girl sitting in a chair and next to her is her sister. And both of them are sitting there playing with their toys. And then mom is on the exam table and you go all the way to the other side and there's the doctor sitting in a chair. Looking away from the rest of the family typing on his computer.
Catherine Price:
Hmm. Wow.
Dr. Pierre Elias:
And what happens when an entire generation of people grow up and that's their vision of what it's like to interact with your doctor?
Catherine Price:
Hmm.
Dr. Pierre Elias:
And I think that speaks to some of the challenges that we're facing right now is it doesn't feel like technology is in service of medicine.
Catherine Price:
Mm-hmm.
Dr. Pierre Elias:
And so I think, both healthcare providers and patients deserve better technology, and that's really the guiding light for all of this work.
Catherine Price:
Okay. I mean, it makes total sense from both a patient perspective and then from the physician perspective.
So, I'm really curious about understanding how you came to be doing what you are doing now. Specifically in terms of how you got into data science and then started to combine these two fields. So, can you tell us a bit about that?
Dr. Pierre Elias:
I always found medicine interesting, but I always know I needed something a little different.
It would, like, the traditional path wasn't, wasn't quite for me. I went to Duke for medical school and your second year is your kind of first clinical year in the hospital and then everyone has kind of a year off for other scholarly pursuits. And that was something that really appealed to me to kind of scratch this itch and better understand what else did I want with medicine instead of just a more traditional route.
And I ended up going to California UCSF to work with Bob Wachter, who's now the chair of medicine at UCSF. He was the one who said, listen, you know, if you wanna make healthcare better do it through technology. And so that actually led to me doing an internship at a data science company in the Bay Area.
Just imagine, you know, a startup in an office park, you know, right outside of San Francisco, literally, everything's a whiteboard. The walls are whiteboards, the tables are whiteboards, everything's a whiteboard.
But we were building a new way to represent medical information. So anytime you type in a medical diagnosis in Google, you get this little box on the right-hand side. We were the people who created the first version of that. And so that was a really exciting experience.
And I still, you know, remember thinking through, oh, how do you take a huge amount of data and condense it into something that's meaningful and usable for the world? But I decided that I wanted technology to be in service of care and that told me I needed to go back and finish my medical training.
Catherine Price:
And what do you mean by that? Technology in the service of care?
Dr. Pierre Elias:
Technology, a lot of times can be a means to an end. So, I could've had that job and then I could've just gone and been a healthcare data scientist and that life is a pretty great life. But I think it's easy to get separated from the end problem and the person who's really affected by what you create.
So, you become really separated from, is this technology that I'm creating, actually solving a problem that matters or not. And knowing are you solving a problem that needs to be solved is a surprisingly difficult thing.
Catherine Price:
Mm-hmm.
Dr. Pierre Elias:
Even to this day, it's one of the things I spend the most time developing. You know, probably for every 10 ideas we come up with, we pursue one. Cuz we're like, that's actually a problem that technology can uniquely solve and is worth pursuing.
Catherine Price:
Okay. So, to fast forward just a bit… so you now run a lab at Columbia that's dedicated to doing that, right? So, can you tell me about CRADLE?
Dr. Pierre Elias:
So, CRADLE is the cardiovascular and radiologic deep learning environment. So that is the lab that I run. I am a co-investigator with two phenomenal people. Tim Poterucha is another cardiologist here at Columbia University and NewYork-Presbyterian, and Chris Haggerty, who is a PhD data scientist.
And the three of us have kind of built this collective that we call CRADLE, which is the place where we develop, validate, and deploy deep learning technologies to improve cardiovascular health.
One of the areas where we particularly focus on is how do you take cheap diagnostic testing and get more information out of it.
Catherine Price:
Okay…
Dr. Pierre Elias:
So, where's the problem here? So, the problem here is that echocardiograms are expensive. They're anywhere from $600 to $3,000 and they're usually symptom driven. That's the most, like, that's the most likely reason why you're gonna order one. So, you couldn't just order an echocardiogram on every person.
Catherine Price:
Because how much does that compare? What's the cost comparison to an ECG?
Dr. Pierre Elias:
An electrocardiogram is probably 50 bucks, maybe less.
Catherine Price:
Wow.
Dr. Pierre Elias:
Well, so, you know, it's, it's orders of magnitude, difference in cost and just in prevalence. Right? So, we do 10 electrocardiograms for every one echocardiogram. And like I as a cardiologist I can look at electrocardiograms, I can diagnose certain diseases, but there are other ones that I would never be able to diagnose just looking at an electrocardiogram.
But could we develop algorithms that can kind of look beyond what a cardiologist can and see is there a reason that someone should get an echocardiogram, a more expensive test or ultrasound of the heart?
Catherine Price:
Hmm.
Dr. Pierre Elias:
So, we asked ourselves, you know, can we find a way to determine who needs an echocardiogram before they develop symptoms, ideally, or at the very least, if they have symptoms, make sure the right people get funneled to the right test.
Catherine Price:
Mm-hmm.
Dr. Pierre Elias:
And if we can determine of all the people who have electrocardiograms, who needs an echocardiogram and do that for every electrocardiogram, whether it's a patient being seen at like a community hospital, a patient being seen at a big academic center… if you can run an algorithm and say, “Hey, there's something wrong here. You should get some more testing on this patient.” That could be something that would be really valuable and could allow us to make better use of the cheap diagnostic testing we already do today.
Catherine Price:
Huh. So, I mean, that makes a lot of sense. So, I’m wondering, as a tool, why is deep learning so well suited to evaluate imaging like electro and echocardiograms?
Dr. Pierre Elias:
Medical Imaging is a place where machine learning and deep learning can make a big, big difference. So, one, medical imaging has just had human interpretation. The human eye is amazing, but it has very clear weaknesses. Just look at all the optical illusions that exist, right? So, there's forms of pattern recognition we're very good at, and other forms of pattern recognition we are not good at. Second, just a ten-second electrocardiogram, the classic one that we do is 30,000 data points.
Catherine Price:
Huh.
Dr. Pierre Elias:
Right? So imagine how many pieces of information are just kind of being left on the table there. So, we have a lot of information there. But for the first time, we now have medical technologies that can actually really assist us and find gaps in our understanding and knowledge from medical imaging. So that's why this is such an exciting time and this is why I think medical imaging and machine learning are a really good match.
Catherine Price:
I mean, I’m really stuck on the 30,000 data points. That is a lot of data. But I mean, in general, where are you actually getting the ECGs and the other data that you are using for CRADLE?
Dr. Pierre Elias:
That's a great question.
You speak to my heart–a data nerd at the end of the day. One of the big challenges in medicine right now is you wanna create meaningful information and you want it to be translatable beyond your doorstep, and the only way you're gonna be able to do that is by having many different doorsteps.
Catherine Price:
Huh.
Dr. Pierre Elias:
We are at an incredible institution. So, if you think about NewYork-Presbyterian, we have two major academic medical centers and a number of hospitals across the greater New York area. There's no microcosm that looks like this basically anywhere else in the world. And that's a big part of why I think NewYork-Presbyterian is able to do this sort of work.
We have huge amounts of data and what we've really done is we have collected and curated millions of cardiac images. So, echocardiograms, electrocardiograms cardiac catheterizations, you name it, we have access to it. Both the medical images themselves, the reports and interpretations by clinicians over, you know the course of 30 years, really, you know, some of our electrocardiograms go back to 1985.
Catherine Price:
Wow.
Dr. Pierre Elias:
And so, we’re able to tell stories and quantify things that have only been qualitative before in a way that just hasn't been doable in medicine until now.
Catherine Price:
So that's interesting. So essentially, you need to have excellent data from a variety of different sources. The next step would seem to be to identify specific problems you can use that to solve using machine and deep learning.
Dr. Pierre Elias:
Yes, exactly. So I'll never forget being in the cardiac ICU overnight and having a patient transferred to me. This was a man, no past medical history who had shown up at a a community hospital emergency department a few months before with chest tightness and shortness of breath.
They did a workup overnight and they said, listen, you're not having a heart attack, but you know, when I listened to you with a stethoscope, you have a murmur on exam. So, you should just go see your primary care doctor about the murmur, but you’re not having an heart attack. Everything's okay.
Life got busy. He didn't make it to that primary care doctor appointment, and then three months go by, he shows up in that same emergency department with the same symptoms, chest tightness, shortness of breath. Although this time he's in full on respiratory distress, he has to be put on a ventilator. And they do an ultrasound of his heart and they find out he has severe valvular heart disease, specifically severe aortic stenosis. And Columbia is a world leading center in the treatment of valvular heart disease. And so, he's kind of immediately transferred to us.
Catherine Price:
Wow.
Dr. Pierre Elias:
They're like, “Hey, he's really sick.” And I remember he came overnight and he was in multi-organ failure, and I worked on him all night long and we talked about it with our multidisciplinary valve team and he ended up dying that week.
Catherine Price:
Oh wow.
Dr. Pierre Elias:
And this is someone where if we had just known he had severe valvular heart disease, he could have had a same day outpatient procedure. And he'd be alive today.
Catherine Price:
Wow.
Dr. Pierre Elias:
So what I really took away from that is you can't treat the patients you don't know about.
Catherine Price:
Mm-hmm.
Dr. Pierre Elias:
And that really kind of set me on this course of how do you find these patients at the right time and get them to the right care?
Catherine Price:
Okay.
Dr. Pierre Elias:
So these are conditions where they can often go missed until very late in the disease course because patients often develop symptoms late in the disease course. But the sooner we know about your valvular heart disease, the sooner we can start taking care of it.
Catherine Price:
Mm-hmm.
Dr. Pierre Elias:
And we can delay progression of it. And then also we wanna make sure that the symptoms, when you do develop them, they're extremely vague. Think about that patient I told you about, some chest tightness, some shortness of breath that could have been caused by 1,000,001 things, but we need to know that it's caused by valvular heart disease in order to get him to the surgical treatment that he needs.
Catherine Price:
Okay. So that’s what you’re trying to do with the ValveNet project right? Like, that actually helps you detect valvular heart disease. So, can you tell me about that?
Dr. Pierre Elias:
So, the point behind ValveNet is to have a system where every electrocardiogram done across the health system in real time has useful technology looking at it so we can find the patients who need additional care, regardless of where they show up.
So, you know, I as a human expert, can't detect valvular heart disease from an electrocardiogram. So, if you gave me a stack of ECGs and you said half of them have valvular heart disease, half of them don't, tell me which half are… I’d bat like 52% or something like that. Like maybe a little better than random chance, but not much.
But we asked ourselves, could artificial intelligence models, particularly machine learning and deep learning models, looking at the electrocardiographic waveform, could they do that?
So, we took all of the patients who had ECGs and echoes in our database and we said, okay, this echo was done within a year of the ECG.
And we trained a model and we said, all right, you're gonna learn from all these electrocardiograms and you're just getting basically the electrocardiographic waveform, their age and sex. There's no way to cheat. Because these models love to cheat. They are the slimiest person you've ever met. They love to find ways to cheat.
Catherine Price:
What do you mean by cheating? What are these machines doing?
Dr. Pierre Elias:
So like, you know, some of our projects, we use chest x-rays, but if you use all chest x-rays, portable and upright films, the portable films are done on inpatients. And the model can immediately tell the difference, and it can be like, well, that person's sick because, they're not healthy enough to go to an outpatient center and stand up for a film. So, I already know something's wrong with them.
Catherine Price:
Huh.
Dr. Pierre Elias:
So, you spend a lot of time making sure that's not the case.
Catherine Price:
So, how do you prevent ValveNet from "cheating"?
Dr. Pierre Elias:
So the first thing is, minimize the total number of pieces of information. So really all that this model gets is just information that's on the electrocardiogram. So it gets their age and sex and the electrocardiographic waveform. So ventricular pacing would show up on an electrocardiogram.
We removed all of the patients with ventricular pacing because that means they already have a pacemaker. And you could imagine that's a big boost to the models. Like, oh, well they've got a pacemaker, there's already something wrong with their heart.
Catherine Price:
Mm-hmm.
Dr. Pierre Elias:
So, maybe there's more that's wrong with their heart.
Catherine Price:
Huh.
Dr. Pierre Elias:
So, you do a bunch of tests like this, right? You say, what are the things that don't have to do with physiology that the model could learn from, and how do we erase those from the data set while still keeping a patient population that we think is representative and accurate?
Catherine Price:
Gotcha.
Dr. Pierre Elias:
And so, from there, what we wanna do is, we trained a model on all of the patients who had electrocardiograms and echocardiograms and said, okay, this is what the electrocardiogram of someone with moderate to severe valvular heart disease looks like. And this is what the ECG of someone without it looks like.
Can you tell the difference? And it's able to do it with quite a high level of accuracy, much higher than a human expert would be able to achieve.
Catherine Price:
Hmm.
Dr. Pierre Elias:
It's able to do that on a test set of patients it's never seen before at our hospital, at another hospital. But does that actually work when you apply it prospectively? I think the only way you know is by going out and testing it.
So, one of the incredible things about NYP is they believe in us. They believe in this work. And so, we're running multiple prospective clinical trials on these different AI models. So right now, ValveNet and some of our other models, they run on every electrocardiogram done in the health system.
And we find patients that the model says is at very high risk of valvular heart disease who haven't had an ultrasound of their heart done. We reach out to that patient's doctor, we say, “Hey, this is what we think is going on.” And we reach out to the patient and we say, “We'd like to enroll you in a research trial.”
You know, “We'll pay for the ultrasound of your heart to be done. We'll arrange transportation, if we find anything concerning we're gonna make sure you have appropriate follow up.” And so we're still kind of in these pilot phases, but we’re able to run these AI systems every day in real time, on every electrocardiogram, find those patients who are at risk for disease and do something about it.
And the first few times that we found undetected disease that needed to be acted on... I mean, I cried.
Catherine Price:
Oh wow.
Dr. Pierre Elias:
It was this moment of joy, but also this moment of relief of… I don't know how well this is gonna work, and I still don't know if this is gonna truly, positively impact clinical care in the future, but it does work. It does do something I, as a human expert, can't.
Catherine Price:
Wow.
Dr. Pierre Elias:
Because I, you know, we will review the chart and I'll look at the electrocardiogram and it's not like it's obvious to me in retrospect, you know, there's a lot of people who are sick, and if you really took all the people who are sick and say, okay, tell me which ones actually have this disease, or need an echocardiogram specifically, I still wouldn't be able to tell you.
So that to me is still pretty astounding.
Catherine Price:
That's really amazing. And it makes me wonder, there's a number of ways to interpret the story you just told, and one of them is like, oh, well this deep learning algorithm caught something that human doctors didn't, so…is it gonna replace human doctors? But then there's another version of that where there's some complementary relationship that the two could have.
And I'm wondering if you could speak to how you see this ideally working in the future?
Dr. Pierre Elias:
Listen, I don't think AI or robots are gonna be replacing doctors anytime soon. Doctors are immensely necessary to this whole process for a number of reasons. So, one, there's a ton of problems I don't want a robot going near. At all, right?
So like, should you put this transplanted heart inside of someone or not? Let's maybe not trust the robot for a long time with that. Right? There's a lot of ways that these, you know, machines can incorrectly interpret information and so I still want a human driver in the seat for a lot of the complex high-risk decisions that we have to make.
Moreover, doctors are still humans and I think sometimes we expect inhuman things of them. And so, doctors are really good at managing the patient and being there for you and navigating illness with you.
But they shouldn't have to be people who instantaneously memorize never-ending lists of problems or double-check, did you actually get that medication that I sent to the pharmacy?
There are all of these things that shouldn't be a doctor's problem that are important for medical care. And lastly, it's okay for us to say they have a visual cortex that is incredible at certain things and has limitations in other things, you know?
It's okay for a car to be faster than a human on a bicycle, right? You're not judging the human for that and saying, oh, get rid of all the humans. So let's just make it so that you can spend your day doing the complex things that I want a human to do, and you've got a machine that's able to assist and do things you otherwise can't.
We already do this. It's not like I can take a vial of a patient's blood and then say, ah, their sodium is this, their troponin is this. Right? We already do this. But I think there is like healthy skepticism from clinicians, which is just like, is this gonna make my life harder or worse?
And I remember talking with a colleague and she said, I just don't see how this is going to help me make the decisions I need to make for my patients. And I turned to her, and I said, frankly, I don't think it will because I think you're an exceptional clinician. And I think you… by the time a patient comes to you, it's all hard choices.
What I want is, I want to get that patient to you sooner so that they didn't remain undiagnosed for as long as they did, and you could have started taking care of them sooner. And so I think fully admitting and accepting like, there's a lot of more routine things that need to happen for care to go right, and that's where AI can be really helpful.
Cause the nice thing about these algorithms is if I told you on an electrocardiogram someone had moderate to severe valvular heart disease or a reduced ejection fraction, or a large pericardial effusion, everyone agrees what the right next step is: get them an echo. Right? So you don't have to worry about do you agree or disagree with the AI model, if you believe that it’s relatively accurate, get them the echocardiogram and diagnosis it. And once they have that diagnosis, there are very, very clear management steps that need to happen.
So, all of the things we're looking for, they're not nice to knows, they're need to knows. These are things that are gonna really dictate your clinical treatment.
And so being able to find those medical diagnosis earlier from routine testing is I think something we can meaningfully do with machine learning and deep learning technologies.
Catherine Price:
That's really amazing to think about that you could come into a routine doctor's appointment for something totally different and then, and then have an automated way to catch something that previously would've been completely impossible.
Dr. Pierre Elias:
That's exactly what's happening. Finding out that a sick patient is sick, is important, but the people who were just coming in for like routine testing and being able to get them to care, those are some of the things I'm most excited about. And, just by coming into the NYP system, we have a way to say, “We're gonna take care of you in a way that other people can't.”
It doesn't matter where you show up. You get this extra support and care. You know, the people who are being seen at a referral clinic deserve the same quality of care as the people who are coming up to 168th Street or going over to Cornell. There's no reason that they shouldn't have access to that world class expertise that we have here.
And really this is just trying to distill that expertise and make it available to everyone we take care of.
Catherine Price:
That’s fascinating, yeah. You know, a lot of attention is being paid these days to discrepancies and disparities in healthcare, both in terms of the healthcare that's provided and the access to the healthcare.
And, I was wondering if you could give some examples or tell us about an example of a way in which a deep learning model might be able to actually help correct some of these disparities.
Dr. Pierre Elias:
It's a great question. I like going against the grain a little bit, and so there's a lot of stuff out there right now about how AI is biased.
And don't get me wrong, AI can very much be biased. But, do we sit down and ask ourselves, how biased is current clinical care? And can AI help us with that bias?
So cardiac amyloidosis is this rare cause of heart failure that's probably very underdiagnosed that we happen to be world-leading experts in. So what we did is we looked at what is the racial and ethnic breakdown of people who receive different forms of care.
It's about 40% white, 40% Hispanic, 20% black. Okay, who gets an electrocardiogram? Very cheap diagnostic test. Very similar, 40% white, 40% Hispanic, 20% black. And then who gets a pyrophosphate scan, which is this highly specialized diagnostic test for cardiac amyloidosis, and it goes from, you know, 40 to 50% white to 80% white.
Catherine Price:
Hmm.
Dr. Pierre Elias:
So we said, can we look at a patient population of people who are at risk for this disease and try to make sense of what would an AI model do for this at risk cohort?
And then we said, okay, those patients that the model flagged as potentially positive for cardiac amyloidosis, who are in that highest risk cohort? What is the racial and ethnic distribution of that population? And lo and behold, it goes from 80% white to something like 50% white.
So, if you used AI to help you determine who you should refer for PYP scans… One, you would probably be able to refer a lot more patients cause you would get a true high-risk cohort. And two, the racial distribution of that population looks very different than the racial distribution of who gets referred for PYP scans right now.
Catherine Price:
Hmm. That's really interesting. So, and you're running a trial on this Cardiac Amyloidosis Discovery model too, right?
Dr. Pierre Elias:
Yeah. So for the cardiac amyloidosis discovery trial, we have a couple of different goals here. So one, same thing of serial monitoring of electrocardiograms and echocardiograms to find patients at risk, bring them in, get the pyrophosphate scan, diagnose them and get them treatment for this disease.
And one of the added things we really care about is this racial and ethnic equity in diagnosis. And so one of the benefits that we're hoping to see is being able to increase the number of patients who get referred who are black or Caribbean-Hispanic to amyloidosis clinic. And so, we've actually designed the trial in a way where we're gonna ensure that at least half of all the patients we recruit are black or Caribbean-Hispanic.
Catherine Price:
Wow. Okay. So, it seems like we're just at the very beginning of what might be possible when it comes to this next level of technology and medicine. And I'm wondering if you were able to have a magic wand and sweep it over medicine and achieve what you think might be possible, what would that look like?
Dr. Pierre Elias:
I think it goes back to that child's drawing. So, these are really exciting and meaningful technologies, but like the heart of medicine is, “I'm sick. Will you help me?” And, “I'm here to help you.” I never lose sight of that. And so, the idea here is the documentation burden, it goes away. The assistiveness of the technology is much higher.
And the places where humans may miss things and robots may be better. You know, these unexpected or rare diagnoses or these things that we can't find from cheap diagnosting today, they have assistance.
And so, doctors are not passengers, they're drivers, right? So, they have assistive technologies that have been well developed, that they know how to interact with, and it says, “Hey, I think this person is at risk of this disease, what do you think we should do?” And they say, “Okay, you know, I've been down this road before and so yeah, we're gonna go ahead and get this testing for you.” And I think that’s the magic wand, right? Is all of the parts of clinical care that don't feel like being a doctor go away. And we're like, truly present with our patients. And all of the things that quantitative science can bring to medicine, all of those, those gray zones where we just have to use our judgment and our best guess, we feel like there's a new layer of support to help us guide clinical care. And that's the dream.
Catherine Price:
That's amazing. Well, thank you so much for sharing that vision with us, and thank you so much for making the time to speak with me today. I really, really appreciate it.
Dr. Pierre Elias:
Thank you for having me.
Catherine Price:
Thank you so much to Dr. Pierre Elias for taking the time to share the story of his work and his vision for how data and technology will impact the future of medicine.
I’m Catherine Price.
Advances in Care is a production of NewYork-Presbyterian Hospital. The views shared on this podcast solely reflect the expertise and experience of our guests. To find out more amazing stories about the pioneering physicians at New York Presbyterian, NYP.org/advances.
ReachMD Announcer:
If you missed any part of this discussion or to find other episodes in this series, visit ReachMD.com/industryfeature. This is ReachMD. Be part of the knowledge.
Recommended
Against the Odds: Improving Survival for Children with Pulmonary Vein Stenosis
Against the Odds: Improving Survival for Children with Pulmonary Vein Stenosis
Medical Industry FeatureAgainst the Odds: Improving Survival for Children with Pulmonary Vein Stenosis